In recent years, Artificial Intelligence (AI) has emerged as a game-changer in various industries, including the event industry, revolutionizing the way we work. As a beginner in the realm of AI, understanding the plethora of terms and concepts surrounding AI in events might seem overwhelming but fear not!
In this blog, we will walk you through the essential AI terminologies and concepts, breaking down the complexity to help you grasp the fundamentals and help navigate the world of AI and AI in events which will help you build more eco-friendly and sustainable events.
Before we explore the various terms and concepts, it’s essential to understand the meaning of Artificial Intelligence and AI in events.
Artificial Intelligence and AI in Events
Artificial Intelligence (AI) is a branch of computer science that aims to create machines or systems capable of performing tasks that normally require human intelligence. These tasks include problem-solving, learning from experience, understanding language, and recognising patterns. AI systems are designed to analyze data, draw conclusions, and make informed decisions, much like humans do. One of the essential features of Artificial Intelligence is its ability to learn from data without explicit programming. This enables AI systems to improve their performance over time by recognizing patterns in data and adjusting their behaviour accordingly.
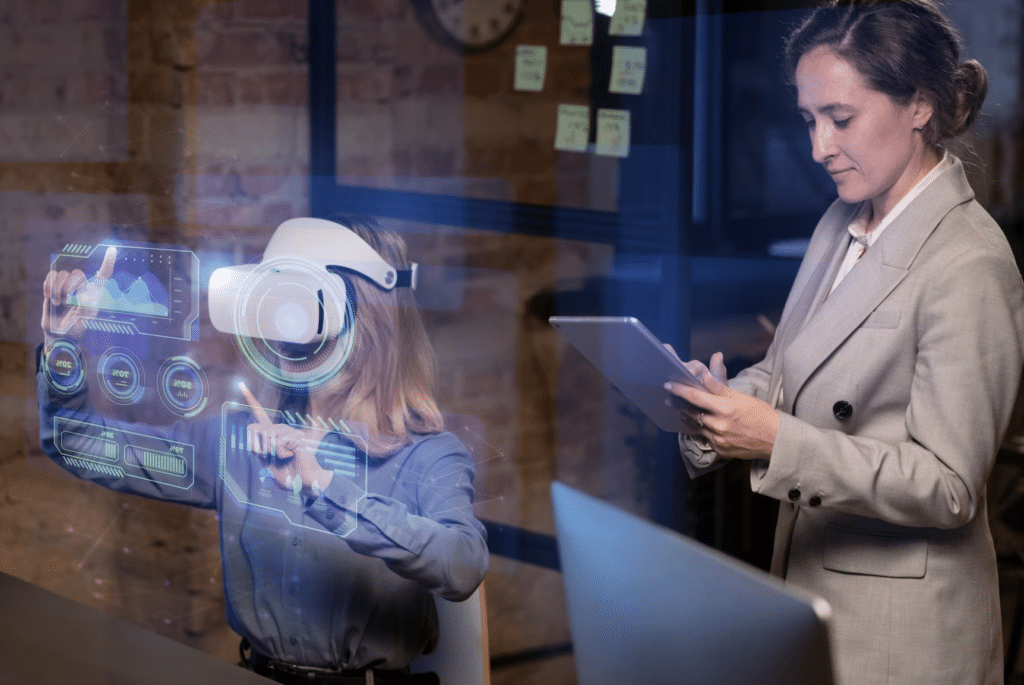
AI in events encompasses gatherings, conferences, and seminars that leverage AI tools to simplify various aspects of event planning. These AI-powered tools can handle tasks such as event scheduling, attendee registration, personalized recommendations for attendees, sentiment analysis of event feedback, and even AI-generated content for presentations or marketing materials.
Now, let’s jump into the various terms and concepts around Artificial Intelligence and AI in events.
Machine Learning
Machine Learning is a branch of Artificial Intelligence (AI) that empowers computers to learn and improve from experience without explicit programming. Through the analysis of data, ML algorithms identify patterns and relationships, enabling them to make predictions, and decisions, or solve complex problems.
The ML process involves data collection, preprocessing, training the algorithm on the data, testing its performance on new data, and deploying the trained model for real-world applications.
Machine Learning algorithms can be broadly categorized into three types:
Supervised Learning: In this type, the algorithm is trained on a labelled dataset, where input data and their corresponding correct outputs are provided. The model learns to map inputs to outputs, enabling it to make predictions on new, unseen data.
Unsupervised Learning: In unsupervised learning, the algorithm is trained on an unlabeled dataset, and it must find patterns, structures, or relationships in the data without any explicit guidance. Clustering and dimensionality reduction are common tasks in unsupervised learning.
Reinforcement Learning: In reinforcement learning, an agent interacts with an environment and learns to take actions to maximize a cumulative reward signal. The agent receives feedback based on its actions and learns from the consequences of its decisions.
Deep Learning
Deep Learning is a subset of Machine Learning (ML) that involves the use of artificial neural networks to process and interpret complex patterns in data. Deep learning models are inspired by the human brain’s structure and consist of multiple layers of interconnected nodes (neurons). Each layer performs specific transformations on the input data and passes it to the next layer, allowing the network to learn hierarchical representations of the data.
The term “deep” in deep learning refers to the presence of many layers in these neural networks. These deep architectures enable the models to handle high-dimensional and unstructured data, making them particularly effective for tasks such as image and speech recognition, natural language processing, and more.
During training, deep learning models adjust the weights of connections between neurons based on the patterns present in the training data. This process, known as backpropagation, helps the model optimize its performance by reducing prediction errors. As a result, deep learning models can automatically learn complex features and patterns from raw data, reducing the need for manual feature engineering, which is common in traditional ML approaches.
Deep learning has achieved significant breakthroughs in various domains, outperforming conventional methods in tasks like image classification, object detection, language translation, and game-playing.
Sustainability Assessment
Sustainability Assessment involves leveraging Artificial Intelligence (AI) technologies and techniques to enhance and streamline the evaluation of environmental, social, and economic impacts of events.
The process typically involves the following steps:
Scoping: Clearly defining the scope and objectives of the assessment, including the relevant environmental, social, and economic aspects to be considered.
Data Collection: Gathering relevant data and information related to the project or policy under assessment. This includes data on resource consumption, emissions, social impacts, economic costs, etc.
Impact Assessment: Analyzing the collected data to assess the potential positive and negative impacts of the project or policy on various sustainability dimensions. This step involves the use of various AI tools.
Comparison and Evaluation: Comparing the impacts of the proposed initiative with established sustainability criteria, standards, or benchmarks to determine its level of sustainability.
Mitigation and Enhancement Measures: Identifying measures to mitigate any negative impacts and enhance positive ones. These measures aim to improve the project’s sustainability performance.
Decision-making: The sustainability assessment informs decision-makers about the potential impacts of the project or policy, enabling them to make more informed and sustainable choices.
Natural Language Processing
NLP is a branch of Artificial Intelligence (AI) that focuses on the interaction between computers and human language. NLP enables machines to understand, interpret, and generate human language, making it possible for computers to process and respond to natural language inputs like text or speech. NLP powers chatbots and virtual assistants, allowing event attendees to ask questions, get information, and receive assistance. NLP can also help event organisers analyze feedback and reviews from attendees, helping event organizers understand sentiments, and identify areas of improvement, and overall satisfaction with their sustainable events.
Key components of NLP include:
Text Understanding: NLP algorithms can analyze and extract meaning from written text, identifying entities, sentiments, and relationships between words or phrases.
Speech Recognition: NLP enables computers to convert spoken language into written text, allowing voice commands and interactions.
Language Translation: NLP facilitates language translation by automatically translating text or speech from one language to another.
Smart Venue Selection
Smart venue selection refers to the use of data-driven and AI-driven approaches to choose the most suitable venue for sustainable events. The use of AI in events involves leveraging various tools and data analytics to make informed decisions that optimize the event’s success and attendee experience.
Key aspects of smart venue selection include:
Data Analysis: Gathering and analyzing relevant data, such as attendee demographics, preferences, and historical event data, to gain insights into the ideal venue requirements. This data-driven approach helps in understanding attendee expectations and tailoring the sustainable event accordingly.
Geospatial Analysis: Utilizing geospatial data and geographic information systems (GIS) to identify potential venues in strategic locations that are easily accessible to the target audience. Geospatial analysis also considers factors like traffic patterns and proximity to public transportation.
Capacity and Layout Optimization: Using technology to assess the capacity and layout of different venues to accommodate the expected number of attendees and event requirements. This ensures that the chosen venue can comfortably host sustainable events without overcrowding or inefficiencies.
Connectivity and Tech Infrastructure: Evaluating the venue’s technological infrastructure, including Wi-Fi capabilities, network connectivity, and power availability. A technologically equipped venue is crucial for hosting sustainable events and facilitating seamless communication and connectivity.
Sustainability Considerations: Take into account sustainability factors, such as energy efficiency, waste management, and eco-friendly practices, to align with sustainable event goals and reduce the environmental impact.
Real-time Data Integration: Leverage AI in events to integrate real-time data sources, such as weather forecasts or transportation updates, to anticipate potential disruptions and make contingency plans for the sustainable event.
Cost Optimization: Analyzing venue costs in conjunction with the event budget to ensure cost-effectiveness and maximize the return on investment.
Attendee Profiling
Attendee profiling involves harnessing Artificial Intelligence or AI in events to collect, analyze, and interpret data about event attendees, enabling event organizers to create more accurate and comprehensive attendee profiles. AI enhances the profiling process by automating data collection, extracting valuable insights, and facilitating personalized event experiences.
Here’s how Artificial Intelligence is used for attendee profiling:
Data Collection and Integration: AI-powered tools can automatically gather and integrate data from various sources, including event registration forms, social media profiles, past event interactions, and other online platforms. AI algorithms can process structured and unstructured data, making it easier to compile a holistic view of each attendee.
Segmentation and Clustering: Artificial Intelligence can segment and cluster attendees based on common attributes, interests, and behaviours. This segmentation helps organizers group attendees into distinct categories, allowing for targeted marketing and personalized event offerings.
Real-time Insights: Artificial Intelligence can analyze data in real-time during the event, providing organizers with immediate feedback on attendee engagement, session popularity, and overall event sentiment. This empowers event organizers to make on-the-fly adjustments to enhance the event experience.
Personalized Recommendations: Artificial Intelligence can generate personalized recommendations for attendees based on their profiles and preferences. These recommendations may include suggested sessions, networking opportunities, and relevant exhibitors or sponsors.
Post-Event Analysis: AI algorithms can conduct post-event sentiment analysis to understand attendee satisfaction levels and identify areas for improvement. This analysis helps event organizers gather actionable insights for future events.
Predictive Analytics
Predictive Analytics is an advanced data analytics technique that uses historical data and statistical algorithms to predict future outcomes or events accurately. By analyzing and identifying patterns in past data, predictive analytics models can forecast future trends, behaviours, or occurrences.
This powerful tool finds applications in diverse fields such as finance, marketing, healthcare, and manufacturing. In finance, it helps predict stock prices and assess credit risk. In marketing, it enables businesses to anticipate customer behaviour and optimize marketing campaigns. In healthcare, it aids in forecasting patient outcomes and disease progression.
By leveraging predictive analytics, event organisers can make data-driven decisions, identify opportunities, and proactively address challenges, leading to improved efficiency and better-informed strategies. However, it’s essential to interpret predictions carefully and acknowledge the inherent uncertainties associated with future sustainable events.
Targeted Marketing Campaigns
Such campaigns leverage Artificial Intelligence to identify and engage specific segments of the audience with personalized and relevant marketing messages. AI analyzes vast amounts of customer data, such as demographics, behaviour, and interactions, to identify valuable insights and group customers into distinct segments. Marketers can then tailor their messaging and content to resonate with each segment’s unique preferences and interests.
AI-powered predictive analytics enables marketers to anticipate customer behaviour and target individuals with the most appropriate offers at the right time. Automated processes streamline campaign execution, allowing marketers to focus on strategy and creativity. Dynamic content optimization ensures customers receive the most relevant content during their buyer journey. Real-time engagement through chatbots and virtual assistants enhances customer interactions and provides timely support.
The result is improved customer engagement, higher conversion rates, increased brand loyalty, and a more efficient and effective marketing approach.
Sentiment Analysis
Sentiment Analysis, also known as opinion mining, is a technique that involves the use of Artificial Intelligence and machine learning algorithms to identify and extract emotions, sentiments, and opinions expressed in text.
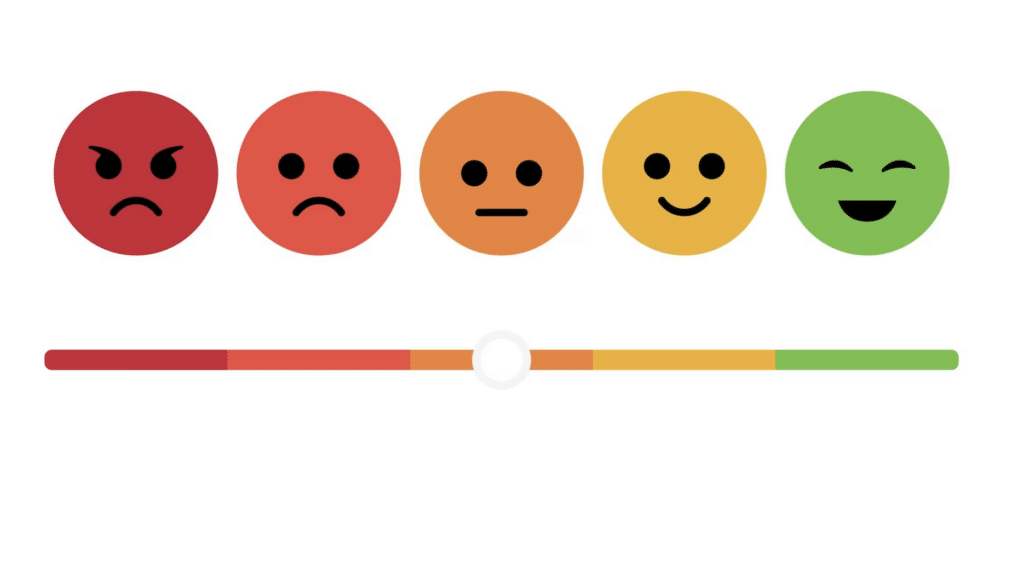
The purpose of sentiment analysis in sustainable events is to determine textual data, such as social media posts, event reviews, attendee feedback, and news articles using such AI in events helps event organisers in understanding the sentiments and opinions expressed by attendees, participants, and the public regarding the sustainable event.
Key aspects of Sentiment Analysis:
Data Collection: Gathering textual data from various sources, such as social media posts, customer reviews, feedback surveys, news articles, and more.
Text Preprocessing: Cleaning and preparing the text data by removing irrelevant characters, converting to lowercase, handling punctuation, and eliminating stop words.
Feature Extraction: Identifying relevant features or words from the text that can be used to infer sentiment. This may involve using techniques like bag-of-words or word embeddings.
Sentiment Classification: Applying machine learning algorithms, such as Support Vector Machines, Naive Bayes, or deep learning models, to classify the text as positive, negative, or neutral.
Sentiment Scoring: Assigning a sentiment score or polarity to each piece of text, indicating the strength of the expressed sentiment.
Aspect-Based Sentiment Analysis: Identifying sentiments towards specific aspects or features of a product or service within the text.
IoT Integration
IoT (Internet of Things) Integration in sustainable events involves incorporating intelligent devices and sensors that are interconnected through the Internet to enhance various aspects of the sustainable event management and attendee experience. By connecting physical objects to the digital world, IoT enables real-time data collection, analysis, and automation, leading to more efficient, engaging, and interactive sustainable events.
Real-Time Attendee Tracking: IoT-enabled badges or wristbands can track attendees’ movements and interactions throughout the sustainable event. Organizers can gather insights into attendee behaviour, preferences, and popular event areas, aiding in event flow optimization and targeted marketing.
Smart Parking and Transportation: IoT sensors in parking lots or public transportation systems can help attendees find available parking spaces or optimize their travel routes to the event venue, reducing congestion and enhancing overall convenience.
Smart Exhibition Booths: IoT-enabled interactive displays and beacons at exhibition booths can engage attendees with personalized content and product information based on their preferences or previous interactions.
Enhanced Attendee Engagement: IoT-driven interactive experiences, such as augmented reality (AR) or virtual reality (VR) attractions, enable attendees to immerse themselves in unique and engaging event content.
Queue Management: IoT integration can help manage attendee queues at registration counters, food stalls, and restroom facilities by providing real-time data on wait times and crowd density, allowing organizers to optimize resource allocation.
Automated Equipment and Services: IoT integration allows for the automation of various event services, such as lighting, sound systems, temperature control, and security, ensuring seamless and efficient event operations.
Artificial Intelligence Ethics
AI Ethics refers to the set of moral principles, guidelines, and considerations that govern the development, deployment, and use of Artificial Intelligence (AI) technologies. As the use of AI in events becomes more pervasive and influential in the industry, it is essential to ensure that its development and implementation align with ethical values and respect for human rights.
Fairness and Bias: Ensuring that AI algorithms and systems are free from biases and do not discriminate against individuals based on factors such as race, gender, or ethnicity. Ethical Artificial Intelligence should treat all individuals fairly and equitably.
Transparency: Artificial Intelligence systems should be transparent and explainable, allowing users to understand how decisions are made and providing clear reasons for AI-driven outcomes.
Privacy and Data Protection: AI applications must respect individual privacy and comply with data protection regulations. Data used for training Artificial Intelligence models should be collected and used ethically, with consent and a clear purpose.
Human Autonomy: Artificial Intelligence should enhance human capabilities and autonomy, rather than replacing or undermining human decision-making and control.
Social Impact: Ethical AI should consider the broader social impact of its applications, addressing potential consequences on employment, education, and societal well-being.
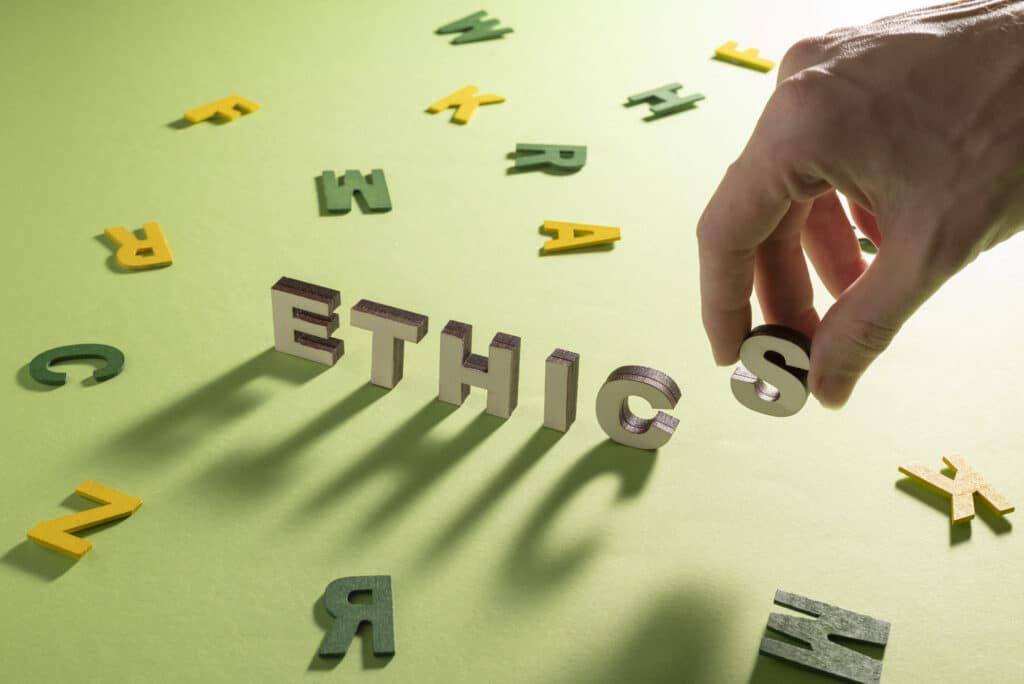
As event organisers embark on their journey into the world of Artificial Intelligence and AI in events, understanding the basic terms and concepts is crucial. This blog has tried to offer a beginner’s guide to AI in events, covering everything from Artificial Intelligence and Machine Learning to Predictive Analysis and Sentiment Analysis.