In the fast-paced world of event management, staying ahead of the curve is paramount to ensure your competitiveness and enhance your capabilities as an event organizer. While the basics of event management are essential, leveraging advanced AI tools can take your event planning and execution to new heights. The thought of employing complex AI tools in your event management processes may be challenging and even more daunting at first – both cost and non-cost factors in using this new platform successfully may be hard to tackle.
Fret not, for you’re in luck. In this article, we will explore a range of cutting-edge AI tools that can help you streamline and enhance your event management processes, ensuring seamless experiences for attendees and organizers alike.
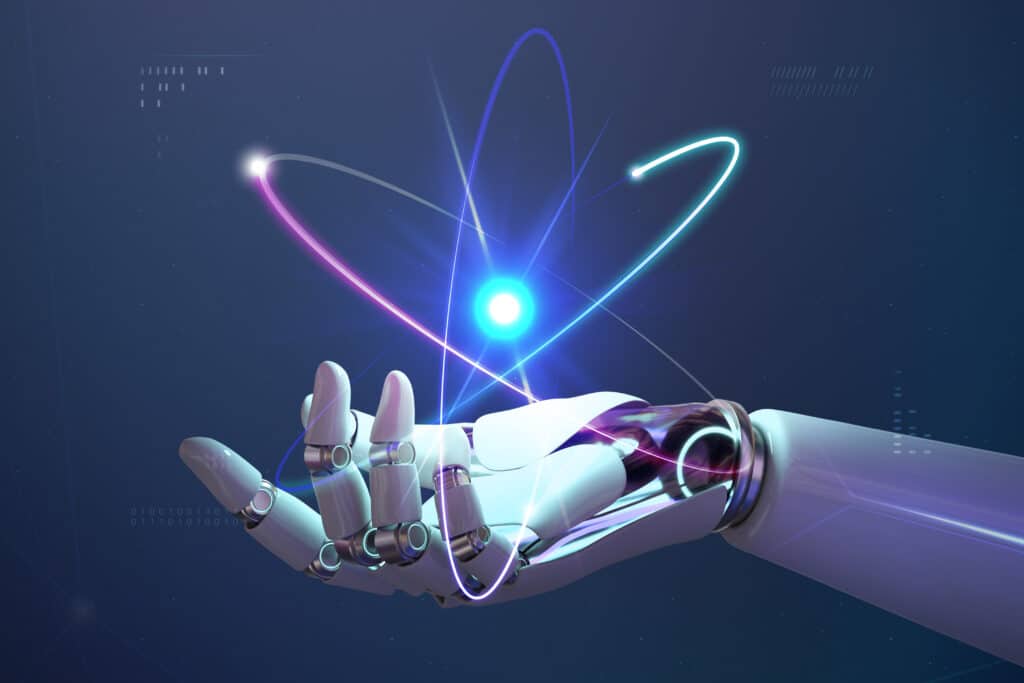
Predictive Analytics
First of all, is one of the most powerful AI tools available for event management: predictive analytics. By analyzing vast amounts of data from past events, attendee behaviour, social media trends, and market insights, predictive analytics algorithms generate valuable forecasts and insights. This helps event organizers make informed decisions regarding venue selection, ticket pricing, marketing strategies, and scheduling, resulting in optimized event planning.
Predictive analysis is an advanced AI technique that utilizes historical data, statistical algorithms, and machine learning to make informed predictions about future outcomes. For example, Catersmith, a Singapore-based company, uses AI algorithms to streamline the process of event catering. Their platform utilizes predictive analytics to recommend menu options, estimate the quantity of food required based on attendee profiles and preferences, and optimize logistics for efficient delivery.
Here are a few key aspects of predictive analysis in event management:
Demand Forecasting:
Demand forecasting is a critical aspect of predictive analysis that involves using historical data, statistical models, and advanced algorithms to predict future demand for a product or service. It helps businesses make informed decisions regarding production, inventory management, resource allocation, and pricing strategies.
The process of demand forecasting starts with gathering and analyzing historical data, including sales data, market trends, customer behaviour, and other relevant factors. This data is then used to identify patterns, trends, and seasonality that can influence future demand.
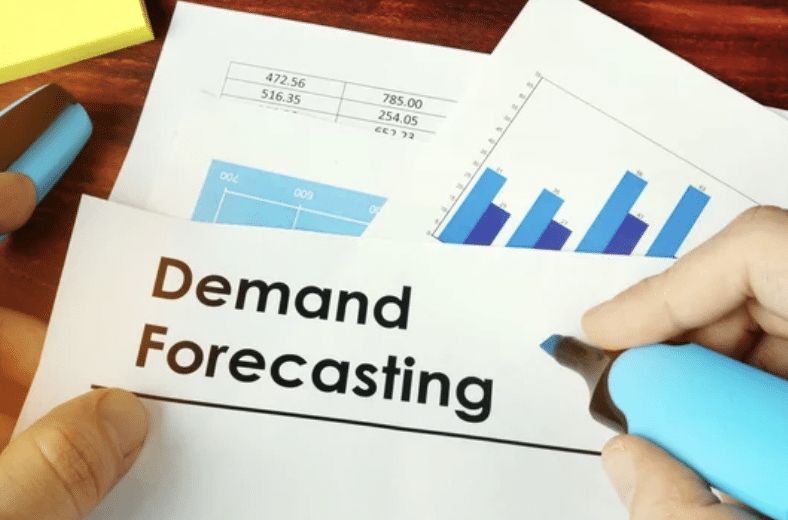
Predictive models and statistical techniques are applied to the historical data to generate accurate forecasts. These models may include time series analysis, regression analysis, machine learning algorithms, and other quantitative methods. The choice of the model depends on the nature of the data and the specific requirements of the business.
Demand forecasting provides several benefits to businesses. It helps optimize inventory levels by ensuring that enough stock is available to meet customer demand without excessive overstocking. It also aids in capacity planning, allowing businesses to allocate resources efficiently and minimize wastage. Additionally, demand forecasting assists in pricing decisions by understanding the price elasticity of demand and predicting the impact of price changes on sales.
However, it is important to note that demand forecasting is not a precise science, as it relies on historical data and assumptions about future conditions. Factors such as market dynamics, consumer preferences, and unforeseen events can impact actual demand, making it essential to continuously monitor and update forecasts based on real-time data.
Ticket Pricing Optimization:
Ticket pricing optimization in predictive analysis is a strategic approach that utilizes historical data, customer behaviour, market dynamics, and advanced analytical techniques to determine the optimal pricing strategy for event tickets. It involves leveraging predictive models and algorithms to forecast demand, assess price sensitivity, and identify the pricing strategy that maximizes revenue and profitability.
The process of ticket pricing optimization begins with gathering and analyzing historical ticket sales data, including pricing information, customer demographics, purchasing patterns, and market conditions. This data is used to uncover insights and trends that can inform the pricing strategy.
Predictive models and algorithms are then applied to the historical data to forecast demand for different ticket prices. These models may include regression analysis, machine learning algorithms, demand modelling, and price elasticity analysis. By incorporating various factors such as event type, date, location, competition, and customer segments, the models can estimate how changes in ticket prices will impact demand.
Based on the forecasted demand, the optimization process involves testing and refining different pricing scenarios. This can be done through simulations, A/B testing, or optimization algorithms to identify the price points that yield the highest revenue or profit margins. The goal is to find the right balance between maximizing ticket sales and achieving optimal revenue per ticket.
Schedule and Resource Optimization:
Schedule and resource optimization in predictive analysis is a methodology that utilizes historical data, predictive models, and advanced algorithms to optimize the allocation of resources and scheduling of tasks or activities within an organization. It aims to improve operational efficiency, minimize costs, and maximize productivity by making data-driven decisions.
The process of schedule and resource optimization begins with gathering and analyzing relevant historical data, including past schedules, resource utilization, task durations, and performance metrics. This data is used to identify patterns, dependencies, and constraints that impact scheduling and resource allocation.
Predictive models and algorithms are then applied to the historical data to forecast future demand, workload, and resource requirements. These models may include time series analysis, regression analysis, machine learning algorithms, or mathematical optimization techniques. By considering factors such as task dependencies, skill requirements, resource availability, and business objectives, the models can generate optimal schedules and resource allocation plans.
The optimization process involves generating and evaluating different scenarios or alternatives based on the predictive models. This may involve simulating different schedules, adjusting resource assignments, or optimizing the allocation of tasks to achieve desired outcomes such as minimizing costs, maximizing resource utilization, or meeting project deadlines.
Risk Management:
Risk management in predictive analysis is the process of identifying, assessing, and mitigating potential risks and uncertainties using historical data, statistical models, and predictive analytics. It involves applying analytical techniques to anticipate and manage risks proactively, enabling organizations to make informed decisions and minimize negative impacts.
The process of risk management in event management typically involves the following steps:
Risk Identification: Identify potential risks that could affect the organization or project. This can be done by analyzing historical data, conducting risk assessments, and engaging stakeholders to identify known risks and potential future risks.
Data Gathering: Collect relevant data from various sources, including historical records, industry reports, market trends, and expert opinions. This data serves as the foundation for predictive modelling and risk analysis.
Predictive Modeling: Apply statistical models, machine learning algorithms, and other predictive techniques to analyze the collected data and identify patterns, trends, and correlations. These models can help predict the likelihood and potential impact of specific risks.
Risk Assessment: Evaluate the identified risks based on their likelihood and potential impact on the organization or project. This assessment allows organizations to prioritize risks and allocate resources accordingly.
Recommendation Engines:
A recommendation engine, also known as a recommender system, is a type of predictive analysis technique that utilizes algorithms and data to provide personalized recommendations to users. It analyzes user preferences, historical behaviour, and item characteristics to suggest relevant and tailored options.
The primary goal of a recommendation engine is to help users discover items of interest that they might not have found otherwise. These items can include products, services, content, or any other items that are part of a larger inventory.
Recommendation engines typically employ two main approaches:
Collaborative Filtering: This approach compares user preferences and behaviours with those of other similar users. By identifying users with similar tastes and preferences, the system can recommend items that these similar users have liked or consumed.
Content-Based Filtering: This approach focuses on analyzing the characteristics and attributes of the items themselves. It creates user profiles based on their preferences and recommends items that have similar attributes or content.
Additionally, recommendation engines can incorporate other techniques such as hybrid models that combine collaborative filtering and content-based filtering, as well as contextual information such as time, location, and user demographics.
Chatbots:
AI-powered chatbots have been all the craze in Singaporean and foreign companies lately. These ‘chatbots’, as the name suggests, are virtual assistants that handle a wide range of tasks, such as answering frequently asked questions, providing event information, guiding attendees to different areas, and facilitating registration processes when prompted. Implementing chatbots powered by AI and natural language processing (NLP) technology is a game-changer for event management. These chatbots operate 24/7, ensuring attendees have access to immediate assistance, reducing the burden on event staff, and improving overall attendee satisfaction.
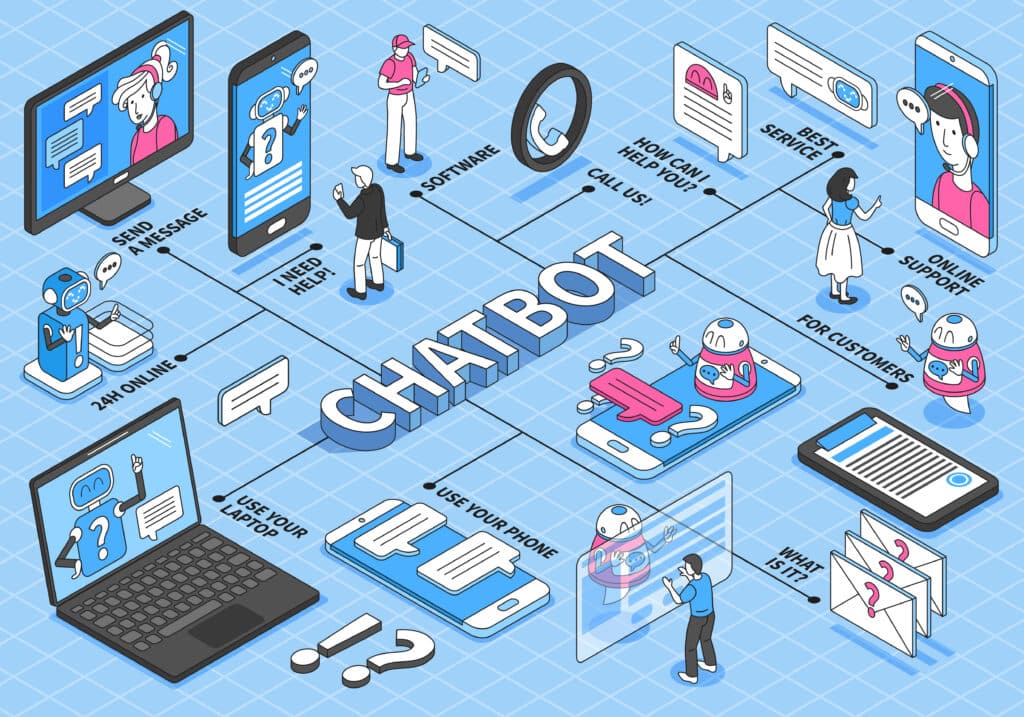
Many Singaporean companies have successfully integrated full-function chatbots into their services, not only streamlining the customer service processes but also making consumers’ experiences more convenient. OCBC Bank, one of the largest banks in Singapore, has deployed an AI-powered event assistant called “Emma” for their corporate events. Emma assists attendees by providing event details, answering questions and providing guidelines and solutions for sessions and networking opportunities. The chatbot utilizes AI and natural language understanding to deliver seamless and efficient event experiences for attendees. As customers enter their queries, these virtual chatbots provide real-time assistance and offer personalized recommendations directly, simplifying processes for both companies and consumers.
How can this be used in the context of event management? Venuerific, a Singapore-based event venue booking platform, incorporated an AI-powered chatbot into its event management platform. The chatbot assists users in finding suitable event venues, providing real-time availability, pricing information, and answering frequently asked questions. By utilizing natural language processing (NLP) and AI technologies, the chatbot simplifies the venue selection process and offers personalized recommendations overall streamlining the event management process.
Facial Recognition:
Facial recognition in predictive analysis is a technology that uses algorithms and machine learning techniques to identify and authenticate individuals based on their facial features and patterns. It involves capturing and analyzing facial images or video frames to extract unique facial biometric data, which can then be compared against a database of known faces for identification or verification purposes.
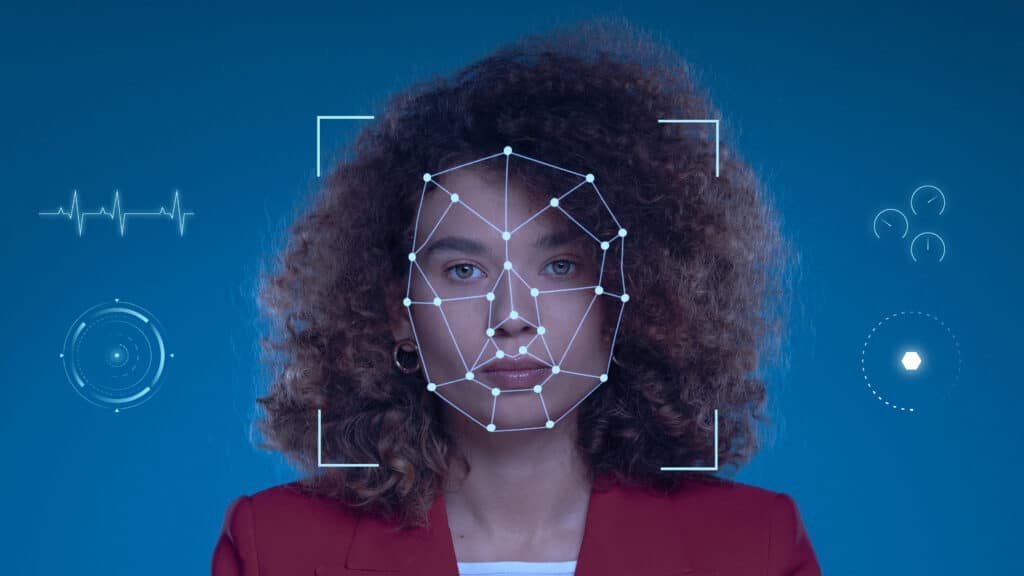
The process of facial recognition typically involves the following steps:
Face Detection: Facial recognition systems use computer vision algorithms to detect and locate human faces within images or video frames. This step involves identifying facial landmarks and distinguishing them from the surrounding environment.
Feature Extraction: Once faces are detected, the system extracts specific facial features, such as the shape of the eyes, nose, mouth, and other distinctive characteristics. These features are then transformed into mathematical representations known as face templates or embeddings.
Database Comparison: The extracted face templates are compared against a database of known faces. This database can include pre-registered faces of individuals or a collection of faces for identification purposes.
Recognition or Verification: Based on the comparison results, the system determines if the face matches any known identity in the database. In identification mode, the system searches the entire database for a match, while in verification mode, it verifies if the presented face matches a specific pre-registered identity.
Facial recognition in predictive analysis has numerous applications across various industries, including security and surveillance, access control, identity verification, customer experience personalization, and law enforcement. It can enhance security by identifying individuals for authentication purposes and monitoring unauthorized access. In customer-facing applications, facial recognition can provide personalized experiences by recognizing and customizing interactions based on individuals’ identities or preferences.
Sentiment Analysis:
Understanding attendee sentiments in real-time is crucial for event organizers to make timely adjustments and improvements. Sentiment analysis tools leverage AI algorithms to analyze social media posts, surveys, and attendee feedback, extracting valuable insights about their experiences and overall satisfaction levels. This enables event managers to identify and address issues promptly, ensuring a positive and memorable event experience for all attendees.
For example, IBM Watson, a leading AI platform, has been employed at the Master’s Tournament, one of the most prestigious golf events. Watson’s AI capabilities were used to analyze vast amounts of historical data, player statistics, and course conditions, providing real-time insights and predictions to enhance the viewing experience for fans and assist broadcasters in their coverage.
Virtual Reality (VR) and Augmented Reality (AR) Experiences:
Advanced AI tools like VR and AR can revolutionize event experiences, whether in virtual or hybrid settings. By creating immersive and interactive environments, these technologies allow attendees to explore virtual booths, attend virtual sessions, and interact with virtual avatars of speakers and exhibitors. This fosters engagement, networking opportunities, and a sense of presence, regardless of physical location, making events more accessible and inclusive.
As the event management landscape continues to evolve, embracing advanced AI tools is no longer a luxury but a necessity. By harnessing the power of predictive analytics, recommendation engines, chatbots, facial recognition, sentiment analysis, and immersive technologies, event organizers can ensure seamless experiences for attendees while optimizing their planning and execution processes. Embrace these advanced AI tools, and take your event management skills to the next level.